This New Manufacturing Technology Gives Your Team Superpowers
Do you want to learn how to use AI to improve your manufacturing quality and efficiency? If so, you need to watch this video of an exclusive event with Retrocausal's CEO, Dr. Zeeshan Zia at the Center for Advanced Manufacturing Puget Sound Innovation Forum. He will show you how his company is changing the game with its no-code AI platform.
In this video, you will see how Pathfinder works and how it can benefit your business. You will also watch a platform demo, hear from satisfied customers, and get answers to your questions from Dr. Zeeshan Zia.
Check out the Innovation Forum.
About Dr. Zia
Dr. Zeeshan Zia, CEO and Co-Founder, Retrocasual
Retrocausal is a fast-growing leader in AI-driven quality assurance systems for manufacturing professionals. Its Pathfinder platform can help you minimize re-work and scrap costs, boost worker productivity, and provide real-time task guidance and analytics.
Website: https://retrocausal.ai/
LinkedIn: https://www.linkedin.com/in/zeeshan-zia-51128398/
About Norman Yu (The Facilitator)
Norman Yu of Kocer Consulting + Engineering facilitated the discussion.
Norman is an Air Force veteran with progressive leadership experience--skilled in problem solving, process improvement, and team building. HIs diverse background has provided opportunities to deliver results in fast-paced, constantly changing environments--from production lines to mountains in Afghanistan. Norman thrives when leading high performing teams, working together to accomplish shared goals.
LinkedIn: https://www.linkedin.com/in/norman-yu-8751b065/
Website: https://kocerconsulting.com/
TRANSCRIPT
Speaker 1 (00:03):
Everyone, welcome to our April Innovation Forum meeting. For those here for the first time, welcome and appreciate the folks that have joined. So, just a bit of background. This is a forum that is gauged to be very interactive, with a lot of dialogue and discussion and collaboration, and is intended for those that have a passion, or a drive to look into innovative solutions, uh, for us to discuss those various solutions and how they can apply to the camps community. So we are honing in on manufacturing distribution in companies, primarily within small and medium-sized businesses. So that's the intent and the vision that we have for this innovation forum. We started this late last year, so we've had a few meetings thus far.
Speaker 1 (00:57):
… and like innovation, we look to improve and iterate as we go. There is a landing page on the CAMPS website with an area where you can submit ideas and feedback so that we can continue to improve and make this as valuable of an hour each month for you as possible. Uh, so please do stay connected for this month. Uh, we are very excited to be joined by Dr. Zhan Zia, who is the, the c e o and Co-founder of Retro Causal, which is a company that leverages artificial intelligence to bring deeper insights for manufacturers, specifically within the discreet manufacturing space. They have an AI software platform called Pathfinder, which Zhan will dig into and show us a demo, which you're very excited to see. And I think what excites me about this is it is all about frontline worker empowerment, giving them insights and real-time guidance and data so that they can make data-driven decisions and empowering the frontline workers to make those decisions. So, I think it's revolutionary. It's very innovative, and I think you'll find some compelling use cases for your manufacturing operation. So without any further ado, I will pass it over to Zun to tell us more about that.
Speaker 2 (02:32):
And Norman, hi everybody. Uh, I appreciate the opportunity. Uh, so, so I'm, I'm Zhan. I used to work at Microsoft for a couple of years and started this company about four years ago. We are based out of, uh, or headquartered in, uh, Redmond, Washington. We also have an office in, in, near Dallas, Texas. And then we have distributors in Mexico and Brazil and Japan. We use cameras and computer vision to help operators avoid assembly mistakes. And we have a bunch of tools to help industrial engineers improve, uh, improve yields and, and quality engineers, minimize, uh, scrap costs and rework costs. And, you know, Norman and Kirk, with your permission, I'll share a few slides. I don't want this to be too, you know, death. Uh, I don't want this to be death by PowerPoint by any means, but, you know, so please stop me in the middle and feel free to ask any questions.
Speaker 2 (03:28):
I don't want to get through a large number of slides. I will show you a couple of videos in the interest of time. I won't bore you with the actual lab. We have a lab and workshop area at our office in Redmond. You are all more than welcome to drop by anytime you want. We have five or six different works stations set up here, right? Actual manual assembly processes that we have mocked up from actual customer deployments in the automotive, electronics, and medical assembly spaces. You are all more than welcome to drop by our Redmond office. Play around with some of those workstations, uh, and, and see some live demos. I'll show you a couple of videos instead of, you know, uh, yeah. So the challenges that we are going after are related to human operators performing assembly jobs.
Speaker 2 (04:20):
It turns out that it's still really, really hard to hire human operators in US manufacturing or find trained labor, especially in US manufacturing. Uh, frequently, uh, manufacturers have to deploy, uh, temporary operators on the assembly line. These folks are relatively less trained, possibly less motivated, and end up doing, uh, this is, and, you know, just in our manufacturing, the number of temporary workers has more than tripled over the last decade. And this is causing an increase in quality issues just due to human assembly mistakes and process improvement. Process design is still done the way it was, uh, basically 120 years ago. Yes, you have the Toyota production system, and yes, you have, you know, lean Six Sigma and all those good things, but it's still very much engineer centric. And I'll talk about, you know, technologies like, uh, chat, G p t or, or, or, you know, stuff that we have built, uh, related to those technologies on how we can use ai, generative AI to improve, to help improve processes as well.
Speaker 2 (05:30):
We have a relatively early-stage company that started four years ago; we have focused more on prominent manufacturers. However, we are keen to get deployed with smaller to medium size manufacturers and learn about their challenges or uniqueness. We, we have just, or we are, we are, you know, in the process of bringing on board a local manufacturing leader to help us basically, you know, get deployed and, and find opportunities within, within the local community. So, uh, there is somebody like that joining us real soon. And, you know, we, we, we look forward to deploying locally. Uh, anyways, the, the solution that we have, we, we give, uh, superpowers to associates and engineers.
Speaker 2 (06:25):
This is purpose-built software to provide human-centric quality control and analytics, right? We do real-time in-process quality control. We provide visibility into new processes. We help you improve processes rapidly, and we help you provide data-driven worker training. Compared to custom solutions, we have internal case studies and still working on getting some of those published. We are much faster to deploy and cost way less than anything else. And our solutions are broadly applicable to a wide variety of assembly processes on the floor. We provide these capabilities, and I'll walk you through a few of them quickly. Uh, you know, our solution is centered on digital work instructions and digital pyo, uh, uh, which help, uh, your auto, you, you know, which help your workers get, get instruction, uh, when they need it, as they need it, right?
Speaker 2 (07:30):
We provide time in motion studies. We record video data for video traceability and have the capability of project guidance. One of our core principles is worker privacy. So, while yes, we are recording, uh, video data of manual assembly processes, we have several capabilities that are centered around maintaining worker privacy. So we don't want to be big brother, and we don't want to be complaining about your workers to you. And I'm happy to dive deep into several things we have in that domain. But let's, let's dive into a demo video. Again, we would love to host some of you at our lab and have you try this assembly process on yourself. This is a mockup of an actual assembly process. Where we are deployed at, this is for an electronics gadget assembly and packaging.
Speaker 2 (08:27):
So this is the, this is the operator's view. Uh, in this case, we actually put an, put a camera on the operator's head just, just to capture this marketing video. You don't need to have a camera on the operator's head, but, uh, you know, there's a display mounted in front of the operator. There is, uh, a projector is projecting guidance, right, right on the workstation. And there is a light tower deployed here as well. So the operator performs a step, and the system is offering alerts right there on the workstation as to the next step that they're supposed to perform. This is a display that's mounted in front of the operator, and you see that here, the operator is also getting instructions right on the workstation, tighten top and bottom screws. Uh, he's seeing these arrows pointing to where he's supposed to tighten these crews.
Speaker 2 (09:22):
He doesn't have to press a button or anything. The system just automatically detects, and right now, I, I'm sure you can't hear it through the call, but on the display, the system shows that an error has been detected, which is that, that the operator was supposed to tighten the bottom screw, but instead of tightening the bottom screw, his hand went in and he started picking up the lid to tighten the lid on the enclosure. So the system is offering him an alert here, and there's also this light tower that's going red, and that's making a noise on the, this, uh, you know, loud factory floor. And basically the, the operator realizes that, that, that, uh, he had skipped this step, and he goes in and fixes that, and the error message automatically goes away. The system detects that that error has been fixed, and the message goes away.
Speaker 2 (10:09):
And again, the operator keeps performing these steps, and the system is detecting, it's tracking the step the operator is at and automatically offering the next step, and then the next step to the operator. We also, uh, in here, you don't see it, but we also have animations and CAD models that you can render directly on the work station that basically tell you exactly how to do the process. We've had, uh, you know, uh, v VP level folks from big companies come into our lab, and, you know, we, we didn't tell them anything. They would just go to this workstation and, and do this process without talking to anybody, and they would be able to do it completely, you know, uh, autonomously and, and, and successfully. So that's, that's, that's one part of it, right? Where there's a display that's mounted in front of the operator.
Speaker 2 (10:59):
In this case, it's a little bit too big because this, this was aimed more at, you know, exhibitions and so on, but there's a display. There is, uh, a light tower integration, and then there is that, that projector based guidance that the operator is seeing. This kind of an, an experience is really, really simple to build. You can deploy it in a matter of a, a day or sometimes a couple of days. Here's another example where we don't have projector. Guidance projector is really, uh, just, uh, is, is, is, is optional. Again, in this case, you know, there's this display that the operator is seeing. The system is on the left hand side, you see the standard operating procedure. Each of the steps in this process in board, you see the current step that the operator is currently at. We are also the amount of time being spent on each step, uh, uh, and when the operator makes a mistake, those mistakes are going to appear here.
Speaker 2 (12:05):
And we'll just see that he, I think he makes a mistake, makes a mistake, or misses a couple of steps, and the system is going to show him that. So I think now he misses a coup, skips a couple of steps and goes forward, and the system shows him that he's missing these steps. It offers him an audible alert, and then he goes in and he fixes those mistake in real time while getting those, uh, and, and the system is basically, you know, signing off on those fixes and saying, yep, yeah, you got this. This is great.
Speaker 2 (12:40):
Any questions so far, folks? I can obviously, uh, I'd love to show you a bunch of, you know, real use cases of this sort of thing. In, in automotive, we are seeing a lot of use cases where operators will forget to tighten or, you know, double torque, a bolt while forgetting to talk, torque other bolts as they're assembling an engine. Uh, so that sort of thing. Uh, you know, we, we are finding a lot of use cases there that, you know, the, this operator is supposed to, I think, torque, tighten eight different bolts with this, uh, torque gun. And oftentimes they will double torque a bolt while forgetting to torque others that, that, that, that. So this is a real deployment with, uh, with a large auto manufacturer. Uh, this is another one where, where we are deployed on a moving assembly line, and the operator is, you know, moving to the, uh, back of the vehicle, moving to the front of the vehicle. And, uh, you know, again, there's a big display that's, that you can't see here, but you know, if the operator makes a mistake, this, this sort of light goes up. And, uh, we also have integrations to actually stop the line.
Speaker 3 (13:52):
So, Dr. Zia, I have a quick question. This is Kurt, please. I'm very fascinated with the different work environments that you've shown, and I'm sure that everybody's watching this thinking of their own work environment, you know, so the first one was kind of a tabletop, uh, system, but now we're watching people do this, uh, in kind more, more of open environment. So is there a limitation to the type of environment this technology can work in?
Speaker 2 (14:17):
No. Uh, I mean, uh, obviously you want to be able to see the activities that you want to capture with the camera, right? If, if the, so, so there are environments where we have not been that successful at, for instance, when the operator is doing assembly inside of the, uh, vehicle body, right? Uh, that's then it's just hard to find a placement for the camera that will work. But as long as the camera can see it, one thing we often say is, if as a human looking through the camera, you could understand what's going on, chances are that our system can as well, right? Uh, so this is this, this is an example of a really small, uh, electronic device, again, medical device being assembled. And in this case, the camera is placed really close to it, even though the parts are really tiny because the camera is close enough, it is still able to capture it. Wow. Does that answer your question?
Speaker 3 (15:12):
Yeah. Yes. And, and I'm, I'm, uh, you know, as I'm, I'm amazed by this technology and the, uh, artificial intelligence behind it, uh, but when this is getting set up, are they, is it just watching the workers, you know, do it, do the correct sequence correctly? Yeah. Is that how it's learning?
Speaker 2 (15:28):
Yeah. So, so to set the system up, all you need to do is you go in, you define the task, that is you, you, you name the steps in the process. So you enter a bunch of names. If you have e r P or MES integration, the system can just pull in your, uh, your process definitions automatically as well. But the first step is you just need to go in and define the process itself. I don't know, maybe it's, uh, assemble an, uh, a printer, right? Something like this. And you go in a single camera can capture multiple processes. So I'll just say, this is the default process, and, you know, mechanics, assembly, uh, we can, uh, put multiple substeps under a step, and those substeps can be performed out of order. So, so we do give this the operator this flexibility that they can perform certain steps out of order, uh, while certain other steps need to be performed strictly in order.
Speaker 2 (16:29):
So, so the operator has that sort of a flexibility, but basically, you know, uh, it's very simple to define. A process takes you about five, 10 minutes at most. The second step is you go in and you set up the system to record training data. Uh, we, we typically require, uh, you know, a few, a few cycles of the process that's being performed. So you can ba basically, you go to your work station, you set up a webcam, you set up a Windows machine, and then you are able to control this from it, from this web web-based control panel. You can schedule recordings. So you can say that, okay, can you please do the training set rec, uh, record training, data set for training these machine learning models? And my shift is going to start at whatever, 10:00 AM and you, you just need to record till 12:00 PM and you schedule it, and the system will automatically record that training data. So you define the task, you record training data, and then you go in and you basically label one of those cycles, right? So in this case, the engine, uh, no, I can just go and for some reason,
Speaker 2 (18:02):
So, so this would be the electronic assembly process that I was showing you earlier, right? Uh, what you do is you will go in and you just need to label one of those cycles at the level of individual timestamps. So, because you've already defined the standard operating procedure, so, so out of the videos that you recorded, you tell the system that this step place enclosure on, right? Mount starts here, and it goes from here till. So, okay, this is, this is when the placement was completed, and then this is where the operator is picking up the PCB from Trey, and then this is where he is placing the PCB and inclu, you know, or, or, you know, and so on and so forth, piecing the, placing the PCB
Speaker 3 (18:59):
Dr. Question from, from me. But this is does not seem to take a lot of technical expertise.
Speaker 2 (19:05):
That's right. Uh, so, so we have, we have designed this to be very much self-serve. Anybody should be able to set up these processes, ob obviously we offer the training to do so, but that's all you need to do. Define the task, record a couple of, uh, uh, you know, assembly cycles, label one of those examples. And then basically you select the model, uh, the task, and you, you press this button called request training. This will send your video data to our cloud. Uh, if you are a, you know, if you, if you have a bigger deployment, you could do everything on-prem as well, but the models will get trained in the, in our GPU infrastructure, and you'll get them back in a couple of hours. And basically you're able to go to deploy model and deploy on one of your active workstations. So basically takes you maybe a day, maybe maybe a couple of days to set this system up on a, on a single task. We also have capabilities to address work stations where you are doing, where you're assembling, you know, different kinds of, uh, units, right? Different skews, different models of, of a product. But, but, but essentially the workflow is very similar.
Speaker 3 (20:18):
Dr. Zia, I hope somebody else will come in with a question besides me, but I'm fascinated by this. The same person may not be using the workstation as, as before. So there could be a variety of, of shapes and sizes and heights and, uh, different people. Is, is it, is, does that throw off? Uh, what's your technology's doing here?
Speaker 2 (20:41):
No, it doesn't. So, so the system is able to, when, when it's get, uh, you know, when we are training these AI models, the system will generate a lot of, uh, a lot of, uh, synthetic data to, you know, if, if in your training data, the person was left-handed versus right-handed, we are able to basically capture all of that, those kinds of variations. And that that's how the system is able to, you know, cover a lot of those use cases. Certainly there might be something very extreme going on, right? An operator is doing a job in a completely different way, in which case we will miss it. So we do need some amount of standardization. I I, I, I won't claim that we don't need it at all, but, but the system is able to handle a certain amount of variation on its own,
Speaker 3 (21:24):
My goodness. Oh, that's fantastic.
Speaker 4 (21:28):
Dr. I have a quick question here when you're building the steps out for a particular sku, if you have an environment where there's high variability within the same standard SKU subset, do you have the ability to create like a template routing for this to go, and then you just make the special tweaks for each individual sku?
Speaker 2 (21:53):
Yes. Yes, we can. Absolutely, absolutely, absolutely. Happy, happy to walk that, that kind of workflow as well. But absolutely, and, and, you know, uh, if, if you're, if you're able to come down, we can show you a couple of those kinds of first stations that you've set up locally here. <inaudible>,
Speaker 5 (22:11):
I I have a question. Uh, Dr. Z, can you project instructions onto the assembly? I'm thinking of a control panel application where you've a large control panel with very complicated wiring, very pronged error,
Speaker 2 (22:25):
Right? Yeah, we can, so, so we have done a couple of those kinds of deployments where it's, it's, uh, you know, via harness assembly kind of situations. Yeah, so sure. We are able to do that for sure.
Speaker 1 (22:43):
Sorry to interrupt, but just talking about complex processes, can you talk a bit more about the failsafing aspect with Pathfinder and the, the digital PO Oaks, for example, and how that can be leveraged?
Speaker 2 (22:55):
A hundred percent. So the system also allows you to, basically we have, uh, we offer, first of all, uh, services where we can come in and help you integrate with, with existing iot infrastructure. But the system also provides O P C uua connectivity. O P C U A is the standard that if you have at least a more recent device, right? If you have a semantic, uh, uh, you know, Siemens plc, uh, semantic, S seven, or something along those lines, the system can just, with just a bunch of clicks, you can enforce that, let's say a conveyor belt will get stopped, right? So, so those things are just built into the platform, and you can do those kinds of integrations with the rest of your plat, you know, of your, uh, infrastructure very, very quickly.
Speaker 2 (23:42):
So I'll just take five more minutes and let me, let me walk you all through the analytics and trace capabilities, as well as something that we are just about to launch, which is more in the realm of generative AI or chat G P T kind of a service within the platform that we are launching. Uh, you, you, you saw that we are able to measure those timing analytics, right? Those go into the cloud as well. And if you go to, again, on, on the portal, uh, from you, you, you'll log in through your, uh, your id, you can go to the analytics tab and you can select a certain process. So for instance here, uh, we, we went to this, uh, you know, exhibition MDM West in, in Los Angeles in early February, and we were demoing this process there. The, the electronic pro assembly process that I just showed.
Speaker 2 (24:34):
Uh, we drove the whole workstation there with us, and you can see that, you know, uh, 90, we captured 96 cycles in this process. A lot of those were bad cycles. We were just being dramatic and making mistakes for the op, you know, the exhibitors, we see the average cycle time for this process was three minutes, 43 seconds, completely automatically captured that. It has captured that the average value add percentage was really low. Again, we were doing relatively lit, less little work and talking to people more, uh, the tact percentage is relatively low because, uh, the system noticed that, uh, you know, we were, we were on the flow for a long time, but accomplished relatively less, right? The system expected us to do a hundred si uh, units, but we were only building 31 units. Uh, it able to show us, it has measured these step level timings.
Speaker 2 (25:28):
Uh, so for instance, for this step, the expected standard time is five seconds, but we were doing it in 4.68 seconds. And this standard deviation is this, and this on the right side, you can see the cycle time distribution. So you see how many cycles were performed in how many seconds. These are good cycles, you know, the standard cycle time, we put it in as a hundred seconds, but they're also several cycles where the cycle time is more. So I can just click on one of those bars, and it takes me to video recordings of those cycles that it has seen. So, for instance, let's just go here, and this is, this is us really on the, or, this, this is me really on the exhibition floor and showing this to a, uh, to a, to, uh, to, you know, uh, to, to an audience member doing this process.
Speaker 2 (26:23):
The system is playing back the entire process. I can click on any step, and, you know, it has intelligently tagged the whole process. I can also, you know, uh, comment on this and tag my colleague Craig. Uh, you can, you can tag, uh, colleagues, industrial engineering colleagues and tell them, Hey, what's going on? At a certain point in the video, uh, that, that's what we call video traceability. We, we ta uh, you know, we capture, uh, missteps. We capture the amount of non-value add time in across shifts. Uh, we ca again, we capture individual cycle times. And we, you can see also the work that was performed. So we were on this exhibition floor on the seventh, eighth, and 9th of February, and we were there from morning 10:00 AM to 5:00 PM and you can see, uh, you know, every individual cycle represented here by a stick. Uh, I already showed you indirectly the trace functionality, you know, when you, when you looked at these videos. We also have this ergonomics capability where the system can basically perform ergonomic assessments. You can record videos with a smartphone, upload them onto the portal, and just observe, uh, results, uh, ergonomic assessment results for those videos.
Speaker 6 (27:45):
Let's see if I can find one that,
Speaker 2 (27:59):
So again, this is, we, we are trying to build a com holistic process management platform centered on manual work, right? So you'll see that this is an, uh, this, this is, uh, one, one of our colleagues. He's in our, uh, lab, and he's doing an assembly. You know, he's, he's doing something there, and the system is able to extract his skeletal poses, and based on those estimations, it's able to perform, uh, you know, NIOSH recommended rebar and ruler analysis. NIOSH is this research arm of osha, and you know, in here it has identified the high risk intervals in this long video. So you see, if I click on one of these intervals, you see that this is, this is a dangerous action. In the video, it notices that this is another dangerous action. If you are doing this repeatedly, this, this, you might, this might lead to injuries.
Speaker 2 (28:57):
This is another dangerous activity. It, it is offering certain, you know, uh, guidelines. Hey, look, the, this, the neck angle in this video is really bad. You should do something about this. It's actually capturing individual, uh, an, you know, the actual angle of each individual joints up here. So left arm, right arm. So if you want to fill out, uh, you know, ergonomics assessment spreadsheet, you can do that with readings from here. We are also adding a button here where you'll directly be able to download a NIOSH standard report, right? Uh, so we have REBA and Ruler. These are different kinds of standards that NIOSH has recommends for, for ergonomic analysis. All of this is baked into the platform. And then we have a chat, G P t like interface as well. Let me just take one more minute and walk you through that. We are just about to release it.
Speaker 2 (29:51):
So it's still on the test portal. You, you'll see, uh, just take, we call, we are calling it the Kaizen Advisor. So the idea is that now you can plug in our platform. Our platform is trained on hundreds of thousands of industrial engineering and manufacturing engineering textbooks, research papers, and, uh, you know, block posts, and you can train it on your own organizational knowledge bases. If you have e r P systems, QMS is quality management systems, continuous improvement systems, we can train a generative AI on those as well. And of course, it's connected to our, uh, sensor infrastructure camera and, and IOT infrastructure. And then here, I can go in and select the same process that I was talking about earlier. So think of this as a virtual industrial engineer, if you will, right? Which, which knows everything about your processes from the past. So I selected the same process, specified a certain range of times and dates, and I'm selecting the same workstation, and then I say, just advise, right? And in natural language, the system is going to start analyzing that process, right? It's telling me something about cycle time, something about the value add percentage, and then it starts talking about the most problematic steps in that process.
Speaker 2 (31:12):
And, you know, it's going to talk about how we can improve potentially some of those processes, right? So for instance, step 14, 15, 16, pickup mounting screws, pick up a charger and pick up manual. It says the opportunity is to streamline the process of collecting and organizing these components, which are relatively small and easy to misplace. And it suggests that we could use automated dispensers and other systems, right? To, to streamline this step, right? And I can ask other steps, other questions, what about step three? Uh, did you notice any issues with that? Right? And, and it, it, it, it understands step number three. And it says, look, the, the step three was pick up PCB from tray. It does not appear that there are any significant issues with step three, right? And, and some things that you can't find in chat G P T today. The system also deals with video and images and tables.
Speaker 2 (32:09):
So I can say, uh, show me an image for, uh, you know, and, and I can, you know, literally describe the steps. So this was pick up PCB from tray. I could write step three, but let me write, pick a PCB pickup step, and it's going to, you know, pull, okay? Yeah. So it, it was able to pick up, pull out, pull out that step, and it's showing me that this is how that step looks like. Write, pick up pcb. Uh, let me go into an, an a step that actually had problems. So step six had some problems, right? Let me maybe, uh, uh, video of step six being performed, right? And it's going to pull in a video just of that step, right? Uh, and I can see one example video and of, of course, I can ask it to fetch other videos of that process as well.
Speaker 2 (33:04):
So now I'm seeing this tighten top and bottom screws, right? And similarly, or, or, you know, we didn't, we didn't really get numbers up here, right? Was just prescription of how we, we might do things. I can say, uh, I don't know. Give me a list of problem steps, automatic steps for this process, right? And it's going to, so it creates that list. It's connected to our analytics platform, right? It's able to give me the, the observe time, the standard time, the step level, error rate, the standard deviation, right? So I I, I'm able to see all of that. And, and one, one question that I, I, I, I really have fun. Uh, I, I tried it before already, uh, in, in full disclosure, and, and it gave me some really, really cool answer, which was, any guesses why the lid, uh, has, uh, extra clearance, uh, against the enclosure box?
Speaker 2 (34:07):
Uh, quality has been complaining about that recently, right? And you'll see that, you know, it's, it's, it, because it had, it has the names of those steps, it has access to a lot of organizational knowledge from the past. It has read those hundreds of thousands of textbooks and research papers that says, look, it seems that the talk sensor is a, is a recording excess torque applied on these steps? So steps six and and 10 were tighten top and bottom screws, tighten left and right screws, and then I think it's some, uh, I think I saw it somewhere here. So you see over tightening the screws can potentially damage or break the components on the P no. Uh, it can also cause the enclosure to warp or deform, which can affect the fit and final finish of the final product, right? So, so seemingly it's able to connect those, you know, seemingly unrelated dots and, and get us to very interesting conclusions.
Speaker 2 (35:08):
Obviously, I can also go to compare to different time periods. Uh, I won't dwell on this, but let me just, just quickly show you something there, right? So I choose one time period, and then I choose a different time period. So, sorry, I, I don't have, I'm not allowed to share custom data, customer data, and the, this is all the data I have. This is the data that, you know, we, we went to an exhibition and, and we kind of actually worked there for a of days. So, so I'm now comparing the work that was done on the sixth and the 7th of February with the work that was done on the eighth and 9th of February, right? And, uh, uh, tells me the differences suggest that the worker may have struggled with certain steps during the second time period, right? I can, again, uh, can you dive deeper into step three, right?
Speaker 2 (36:08):
I, I can, I can still talk to it and say, Hey, so, so what's going on? Exactly, right? And, and ask for all of those, those interesting things, uh, those data points. Can you plug the, I don't know, step distribution, uh, well, I dunno, side by side maybe. And, and we get this, this sort of, uh, you know, so this is for the first time period, this is for the second time period and so on, right? So in a very chat like way, you are getting access to, uh, to an AI that understands industrial and manufacturing engineering deeply, that potentially has access to your own knowledge base. So imagine you have several factories in one of your factories. Some of your, uh, industrial engineers have run a kaizen event. They've identified an issue, and, you know, they, they're busy fighting fires all day. They don't have the time to communicate that specifically with, with other factories, right?
Speaker 2 (37:05):
What this sort of a tool can do is automatically ping all the other factories, the right kind of workstations and say, Hey, you know what, it seems like something, something was done on that workstation, which my may might apply to you as well, right? So it can make all those seemingly, uh, you know, hidden conne, uh, you know, uh, hard to form connections, but that's, that's something that we are just releasing in, in a, in a week and a half. Uh, but yeah, so sorry for speaking too long. Would, would love to hear questions and so on. Uh, I, I, I realize that, that we have already kinda,
Speaker 1 (37:40):
You know, Dr. Zia, as an industrial engineer, I'm just totally geeking out with that Kaizen advisor. I absolutely love that, and all the different applications for it. That's really, really cool. You mentioned generative AI a couple of occasions today. For those of us who may just be starting our exploration of artificial intelligence, yeah. Could you give an overview of what Gene AI is and how it differs from the other type of ai?
Speaker 2 (38:06):
Oh, absolutely. I love that. Let me show you some fun examples, right? I'm sure you know, even if you, if even if you're not doing manufacturing, some of these will be fun. So, uh, i, I just created, uh, because we are launching this, uh, this generative tool, right? So I, uh, uh, this, uh, Kaiser advisor tool, so just in the morning I was playing with this, I'm creating a marketing video for that, right? And this is a video that I've created. I don't know if you can hear me. I, I dunno if you can hear the, the sound of this.
Speaker 2 (38:46):
You can see this person, right? He's, he's, he's completely fake. You know, this person does not exist in real life. Oh, right? So what I've done is I've taken this, I can, uh, choose a lot of avatars. So this is, this is synthia, this is, this is some somebody else's software. Obviously, I can choose from a long list of folks. I can choose anybody, any, any one of those. And then I write this kind of script over here, and I can also, you know, uh, and the system basically just synthetically creates that person and creates all of the, his voice. Uh, i, I can choose from a very long list of accents and so on. And the system will just cre animate that person, right? That's, that's, that's an example of generative ai. I'm sure several of you have tried out Chad g p t, right?
Speaker 2 (39:37):
You can do a lot of very interesting things there. Just log in real quick, you know, with Chad, g p t, can, I can ask questions like, explain Kaizen to me, like I am, uh, uh, 12 years old and in poem form, right? Create a poem to explain what kaizens are, are to me, <laugh>, you know, look, it, it says kaizen is a word. So cool. It means to always improve, not just in school. It's a way of thinking, a mindset to be, you know, so, so it's, it's able to do these very, uh, really cool things, right? You can, you can ask it to, uh, let me just stop generating, you know, you can ask it. Very open-ended questions, and it's going to generate that, those create a press release for my new product called <inaudible> Advisor, which is a generative AI tool aimed at helping industrial engineers become more productive. We are, uh, startup company based out of Redmond, Washington.
Speaker 2 (41:07):
So I, I just says, I, I, you know, I, I just put that paragraph in there and it's generating a, a press release for me, essentially, right? And then, uh, so that's an example of generative ai. And I'll, I'll, I'll Norman, I'll, I'll come to you, I'll answer you in a second. You know, I, I, I, I, I, I'm just fascinated by these tools that I, I like to just demo them again, chat.open ai.com. Anybody can use that. It's, it's, it's an, it's a, it's a free tool, uh, SY Studio. You can also use that to a certain extent. We actually bought it for $30 a month, but, you know, you can create very nice videos and presentations around that. Uh, but, you know, so, so it generated that press release for me, and then I can talk to it, right? I can say add, uh, update CEO name to Z in the above press release and, uh, update company name to Retro Causal, and it's going to generate it again and now, but, but now it has, it actually put, you know, it's now filling, filling in the blanks, and it's a actually adding retro causal, a startup based in Redmond here.
Speaker 2 (42:22):
It didn't know the company name because I didn't provide it there, right? So it can do all those things. I can also generate some, you know, pictures. So, so, uh, I was just showing it to, we, we are filing some patents, and I was showing this to our patent attorney. This is starry ai.com. It's a totally different app. And, you know, we can do something like, I don't know, uh, uh, what's Claire? Who's your favorite actor?
Speaker 2 (42:49):
Let's just go with Robert Redford for now. Robert Redford. Okay, let's go with him. Robert Redford in front of, uh, Seattle, uh, skyline. I dunno. Let, let, let's see what it generated. It, it, it takes a couple minutes, but again, so there are two kinds of ai, right? One is generative ai and one is predictive ai. Predictive AI is what you know, is where you give the system some amount of data and ask it to, uh, and ask it to, you know, classify it. So you give it a, a dog picture, you give it a cat picture and a ask the system, Hey, what's in it? That's what predictive AI is. It's going to tell you that it's a dog, it's going to tell you it's a cat. In the, in the case of our system, we are able to train these video analysis models where, you know, now the next time the system sees you perform that action, the system is able to predict, oh, you are doing this step or that step.
Speaker 2 (43:56):
Whereas in case, in the case of generative ai, you can ask it a fairly open-ended, re make it a fairly open-ended request, and it can generate text, it can generate video, or it can generate images right, to your liking, right? I don't know, I I could ask Robert Redford being attacked by, uh, I don't know, teddy bear, right? Let, let, let's, let's see what happens this now. Uh, but you know, so, so, so what we have, we are now moving towards, we have added that generative AI capability into our system as well, where it, where you train it over very large knowledge basis, and then basically it's able to, uh, give you that act as, as an, as an expert. Uh, so Chad, G P T, for instance, has already Chad, G P T has already, so, okay. He's not quite getting attacked by it.
Speaker 2 (44:51):
You see that it's not perfect, okay? But, you know, so Chad, G p t, uh, it has actually passed medical exams, right? It has passed medical boards. It has passed the, the bar exam. It turns out that Chad G P t is almost at the level of, uh, intelligence and smarts that a professional would be. I'm sure that in fact, internally we have run some tests. It seems that it seems to pass the exams for a master's level program in industrial engineering already right outta the box, right? You go to charge and ask it questions, it's going to give you a lot of answers. So we are trying to, you know, combine that power with our analytics engine, and by combining organizations', internal knowledge bases, and, you know, trying to generate that, uh, you know, uh, hand you an industrial engineer if you can't afford a very, you know, uh, highly trained industrial engineer, now you can just use our Lean G PT and use that as your industrial engineer. So, again, so, so, sorry, I, I just keep, keep talking too much.
Speaker 3 (46:00):
No, you're, you're not talking too much. This the, we're all, uh, very excited about this. Uh, I'd loved your examples of how, uh, you know, to me, I, you know, I've been watching what Microsoft is doing with co-pilot, and it sounds like you're doing the same thing with your, with your software, is you're injured, you're, you've integrated ai, I mean, where it does videos, it does pictures. You can ask a questions. Uh, you're gonna be actually giving your customers an industrial engineering capability within your system.
Speaker 2 (46:33):
Absolutely tr try trying to trademark Kaizen co-pilot this week. We'll see if it goes through. It seems like Microsoft has just, you know, just trademarked co-pilot with everything, but ab absolutely, you're right, hundred percent
Speaker 3 (46:49):
That was an excellent demonstration. Are there any other questions, Allison?
Speaker 7 (46:54):
I have a question. I'm curious to know your thoughts about maybe not your product, but, developing, using AI to develop a very lean process. So do you think that AI is capable or would it be applicable in a manufacturing environment to show a series of steps and then ask, you know, chat B G B T or something like that to put together the most lean process for me, to build this assembly?
Speaker 2 (47:25):
That is precisely what we are trying to do. Uh, I'm sure that you, if you just went to even open AI's chat, G P T, I'm sure that it's going to show you something that's, that's not completely unreasonable, right? You, you supplied with the right amount of knowledge, uh, you, you tell it, you know, you talk about what it is that you want to do, uh, it's, it is going to be able to generate at least a lot of alternatives for you. They, they, those might not be the most lean version of the same, and, but, but you know, that, that's precisely what we are trying to get at, right? Build a, an engine that can generate entire factories for you, right? You just, uh, like I just generated that press release, uh, generating entire factories should be just as as easy, right? Hey, hey, I want to have a factory where we, I don't know, ge uh, create tires, right?
Speaker 2 (48:19):
What's the best, or, or, you know, with this and this spec, and it should generate all the works stations for you. And, and it should generate all the standard operating procedures for you, and it should generate all the workstation layouts for you. And maybe you specify a certain, some constraints say, this is the piece of land that I have, this is the area that I have, this is the, uh, you know, I don't know budget that I have. Again, that, that would be a little bit, you know, in the future. But, but I'm sure there are a fair bit of things that we can do already. We didn't talk about, uh, some of the work we are doing with Siemens. Uh, they have this engine called process simulate. So you, if you go to YouTube and just search for Siemens Process simulate or Siemens Jack, you, you will know what I'm talking about in five seconds.
Speaker 2 (49:07):
It's a 3D gaming engine, gaming style engine where you can literally design a whole factory floor, right? But today you have to do it manually, tediously, right? You, uh, and, and, and you can animate things as well. You click on a person, you tell that person to pick some heavy load up and then install it somewhere. So you can literally, you know, simulate processes, uh, entire processes in there. And the, the, the, the stuff that we are doing, not, not necessarily just with Siemens, uh, but, you know, uh, with the broader discreet event simulation in the discrete event simulation space, is we are trying to, you know, uh, hook it into the, that sort of a, uh, setup and, uh, automatically generate processes and, and, and then you can actually play with those processes before you ever have to, uh, actually implement them. Right? Give me just one second and I'll, you know, I went to just YouTube.
Speaker 2 (50:08):
Th this is not us today. Uh, this is, this is Siemens, right? But Siemens has these tools called, uh, simulator to, you know, uh, Siemens process simulate. And in here you can, you can actually take, uh, define a, a whole process, click on the person, uh, change the person's type, right? Body shape and so on and so forth, weight, height, and so on, and make the person do certain kinds of processes. And you can extract timing metrics out of it. You can extract ergonomics out of it, essentially. You can design an entire, you know, entire factory. Uh, you can also simulate, uh, you know, robots and, and so on. But, but you, you get the message, right? Uh, but yeah, and, and we are connecting our, our engine to that as well. And again, absolutely,
Speaker 3 (51:04):
Dr. Zia, I'd like to just kind of get a feeling from you about the future, uh, uh, of manufacturing based just on your technology. And it sounds like to me, you're partnering with some of the very big players who are thinking ahead in manufacturing as well. Uh, but with this access to this kind of knowledge and the guidance, the assistance and guidance to associates, but the information that comes up to the engineers and to management, uh, where, where do you see this going for manufacturers?
Speaker 2 (51:33):
Yeah. Uh, so, so, so we'd like to, you know, uh, make or, or, or, you know, the, the way we look at it is, uh, like I started at the beginning, industrial engineering is still essentially done the way it was done 120 years ago, right? Yes. You have Lean Six Sigma and you, you have all those nice tools, uh, but essentially everything is still very much engineer centric, right? You have an engineer, uh, they come into on, onto your factory floor, right? They work there for a couple of years. They, everything is in their mind, yes, they, they'll, they'll write it down into some Excel sheet, but then, you know, your knowledge systems, knowledge based systems are, are getting so much knowledge into them that it's impossible for somebody else to track them, right? That engineer goes away. And now you don't really know why that process was designed the way it was designed.
Speaker 2 (52:25):
And, uh, we think that in a lot of industries, but we are, we are trying to do this for industry, for industrial engineering, manufacturing, engineering, uh, you're going to start taking all of those knowledge bases, right? Automatically, again, uh, essentially uploading that knowledge into an artificial brain and, uh, super, you know, super brain that, that has that, that has learned everything about industrial engineering there is to learn about industrial engineering. And it, you, you plug it into, let's say you are a Toyota, you plug it into your knowledge basis, it knows everything about Toyota there is to know about Toyota, uh, and then you plug it into our sensor suite, right? And that allows it to actually gain real time understanding of what's the, what's happening on the factory floors, what's the cycle time, what's the step time, and then you're able to essentially ask it very open-ended questions and, and, you know, it gives you answers, it, it designs at dire factories for you and, and so on and so forth. Again, I, I, I know this, this sounds, uh, a bit science fictiony, right? But, uh, so that, that, that's why I started out by talking about some less crazy stuff, right? That, that, that we can deploy today. But also, you know, would love to, uh, you know, share access to this, uh, Kaizen advisor piece and get your feedbacks and everything.
Speaker 1 (53:45):
That's amazing. We definitely live in exciting times right now and we're just scratching the surface. So I know we just have a, a couple minutes left, but Dr. Zia, I definitely want to, uh, uh, thank you very much for taking the time, uh, to speak with CAMPS, and just fascinating stuff. So very excited to see, uh, where your technology goes from here. It's already done so much. Thank you again also for the open invitation to come tour your facility and play around with your demonstration. Absolutely. So I know that that invitation's open to, to camps as well. So, thank you very much for that, as well as appreciate the crash course and artificial intelligence. I learned a lot just from talking to you and picking your brain over the last few weeks. Before we do conclude, I wanted to quickly share with folks on the call. If you don't know where the in, uh, innovation forum landing page is at, you'll just go to the main camps webpage. Under committees you'll go to Innovation Forum, and this is where we have information on upcoming meetings. So this was today's meeting. We also had a recorded interview with Dr. Zia a couple of weeks ago, so you can view that right here as well, where he dives into a bit more about generative AI versus predictive AI and the Pathfinder technology.
Speaker 1 (55:09):
You'll also have a link to the innovation library where Kirk and the team are continuously adding articles and videos for anything that you might want to investigate regarding innovation. And again, if there's anything that you might like to see in an upcoming meeting, or if you have a passion project that you might want to explore, our contact information is down here Stacie with CAMPS or myself. And we'd be happy to hear your thoughts and your feedback. Again, this is very collaborative and intended for us to learn as a group for what's applicable to us as small and medium sized businesses. With that, I wish you all a very great rest of your week and keep a lookout for our next meeting, which will be on May 3rd. And again, Dr. Zia, thank you so much for your time and we look forward to staying in contact.
Speaker 2 (56:03):
Same here. We really appreciate the opportunity. Again, please visit us in Redmond. Uh, we'd love to show you a lot of cool stuff.
Speaker 1 (56:10):
Yeah, wonderful. Thank you. Take care everyone. Thank you very much.
Share this
You May Also Like
These Related Stories
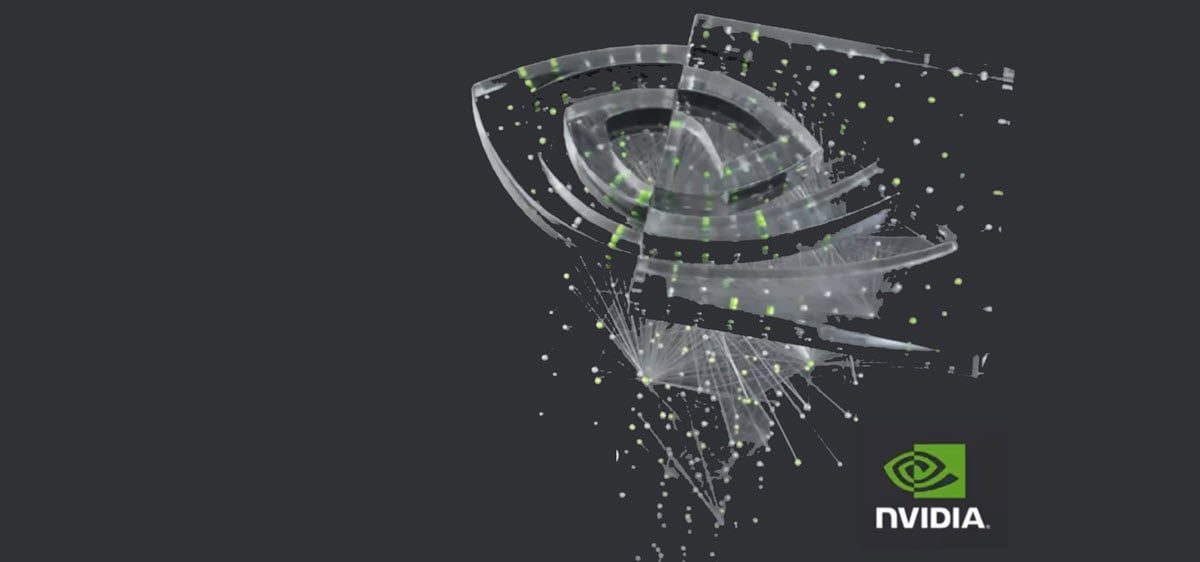
From Simulation to Reality: Advancements in Robotics with NVIDIA's Arsalan Mousavian
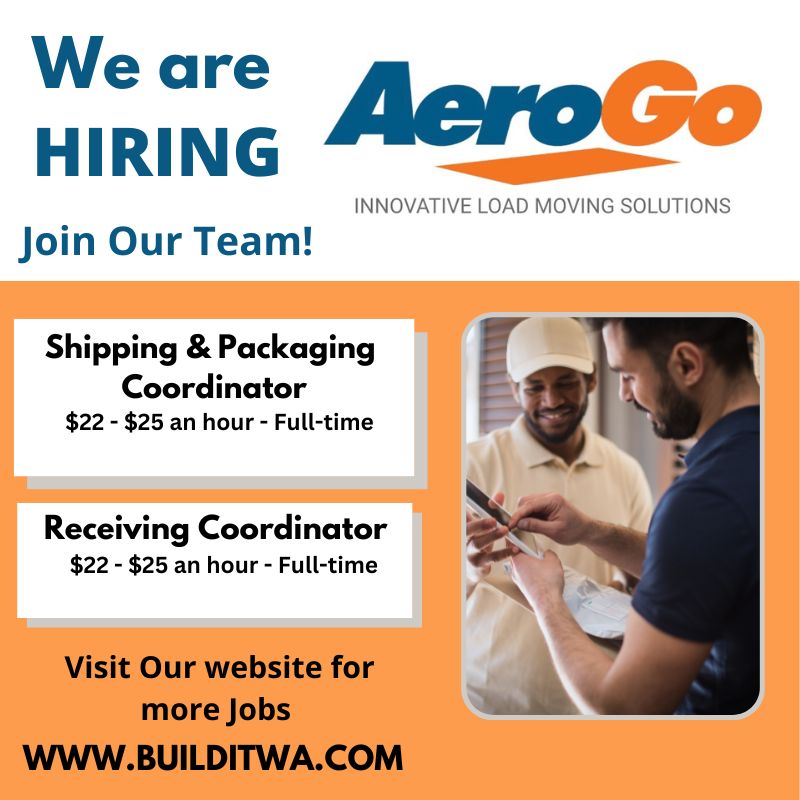
New Job Posting Alert... Explore Opportunities at AeroGo, Inc.
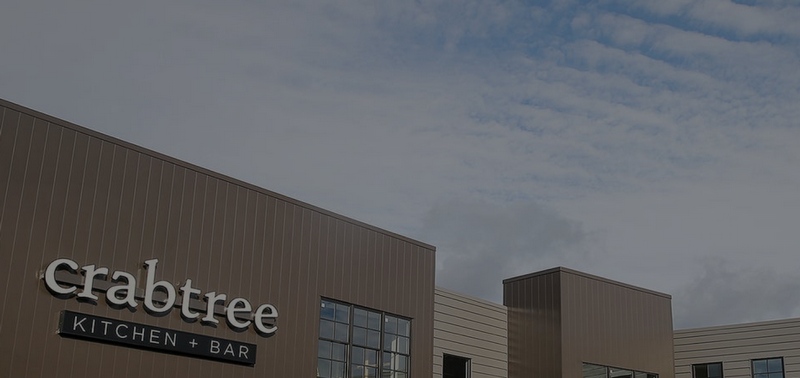
No Comments Yet
Let us know what you think